HEALTH
The Importance of Ethical Machine Learning in Healthcare
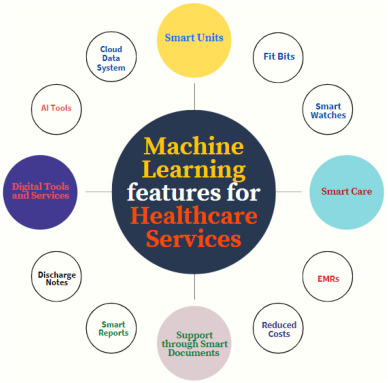
To ensure that healthcare ML works ethically, it must be subject to medical ethics oversight. It is achievable by leveraging the research ethics framework for clinical ML development.
Physicians have two essential qualities that algorithms lack: empathy and benevolence, which are critical for establishing trusting patient-physician relationships. This article adds to the ongoing discussion by exploring these issues through the lens of professionalization theory.
Transparency
Transparency is a critical component of ethical machine learning in healthcare. Following several high-profile cases in which ML has been linked to other concerns about ethical machine learning, there is a demand for a more deliberate approach to AI. It is reflected in activism from civil society, the emergence of over a hundred sets of AI Ethical principles globally (Linking Artificial Intelligence Principles), and government moves worldwide to regulate AI.
However, defining what constitutes ethics and developing ethical ML systems is more complex. Ethical principles often operate at a level of abstraction that makes them more universal, but the specifics of how they apply to any given socio-political context must be negotiated. Moreover, there are often trade-offs between different principles – for example, collecting more data about non-typical users to better tailor solutions to them (for fairness purposes) could come at the expense of privacy.
Fortunately, health care is one of the few sectors with a rich history of addressing ethical risks – a tradition proving helpful in developing practical, transparent ML tools. Leaders in the industry should take inspiration from this work, identifying the potential human rights impacts of their products and providing access to redress for those whose rights are violated by these technologies. In addition, they should consider the possibility that their algorithms may be biased and develop ways to identify and mitigate this risk.
Fairness
Fairness – treating all individuals equally – is critical in healthcare, including ensuring equal access to high-quality healthcare services and preventing discrimination against patients with different social statuses. It can be accomplished by implementing equitable AI-driven healthcare systems that are free of biases and provide accurate diagnoses.
Biases in ML can originate from various sources, including the data used to train algorithms (data bias) and inherent design or learning mechanisms in the algorithm itself (algorithmic bias). Fairness in healthcare requires identifying, mitigating, and controlling these biases so that all patients receive equitable treatment.
A key aspect of fairness is respecting individual autonomy and avoiding coercion or other forms of manipulation in gathering, processing, and disseminating personal data. It is an important issue when using ML in healthcare, especially when collecting sensitive information such as health records.
For example, it is unethical to use ML to determine a patient’s eligibility for a specific service based on their age, disease or disability, political affiliation, creed, ethnicity, gender, social standing, or sexual orientation without explicit consent. Similarly, using a person’s location or hardware to deliver a tailored service is unethical. These ethical issues are often overlooked when using ML in healthcare, but they are essential to maintain patient trust and improve clinical outcomes.
Accountability
ML systems should be held accountable for their decisions and the impact of those decisions on people. The key to accountability is transparency; a clear explanation of how the system makes its choices should be provided. It is essential for both human users of the system and the organizations who develop and deploy it. Ultimately, ML systems should be transparent enough that humans can interpret them, allowing for better oversight, impact assessment, and auditing.
Another accountability aspect is mitigating bias and fairness in machine learning. Bias in training data can perpetuate and even amplify existing biases in deployed models, which significantly impact people’s lives (such as hiring or credit scoring). It is particularly concerning when it comes to healthcare, where misdiagnosis can potentially lead to life-threatening consequences.
While the ethical concerns of ML in healthcare are new, many of the solutions aren’t. These issues are not very different from the medical ethics and research standards that have existed for centuries – from the Hippocratic Oath to ethical guidelines for clinical trials. These are all about ensuring that outside accountability is in place and, ultimately, people can trust the healthcare system to keep them safe. Whether these principles are achieved through laws, policies, or best practices, healthcare organizations must take them seriously and ensure that external accountability measures are in place.
Privacy
Privacy is a critical element of ethical machine learning concerning the data used in training and model outputs. Using sensitive personal information without an individual’s consent can lead to harm, including discrimination, monetary loss, damage to reputation, or even identity theft. These harms can be especially severe in healthcare, where data collection is often tied to specific treatment outcomes for a patient.
Moreover, if a clinician and an algorithm disagree on a diagnosis, the clinician will likely be asked to justify her decision. It puts the clinician at a greater risk of being held responsible for causing harm to a patient compared to an adversarial situation between humans. This problem is not new to medicine or research and has long been a concern of the Hippocratic Oath.
Several academic studies have developed frameworks to help researchers address the ethics of ML in their work. However, many questions remain to be answered, particularly around the scope of these frameworks. For example, having a set of ethical principles that can be used in a broad range of socio-political contexts may be essential. Still, the definition of these principles will depend on local conditions and must be negotiated. Furthermore, it is crucial to consider how to balance different issues in the context of a given project (for instance, collecting more data about non-typical users for fairness purposes might come at the expense of privacy). The bottom line is that ML can profoundly impact people’s lives and should be designed to minimize potential harm.
HEALTH
Doctorhub360.com Neurological Diseases: Advanced Care with AI & Telemedicine
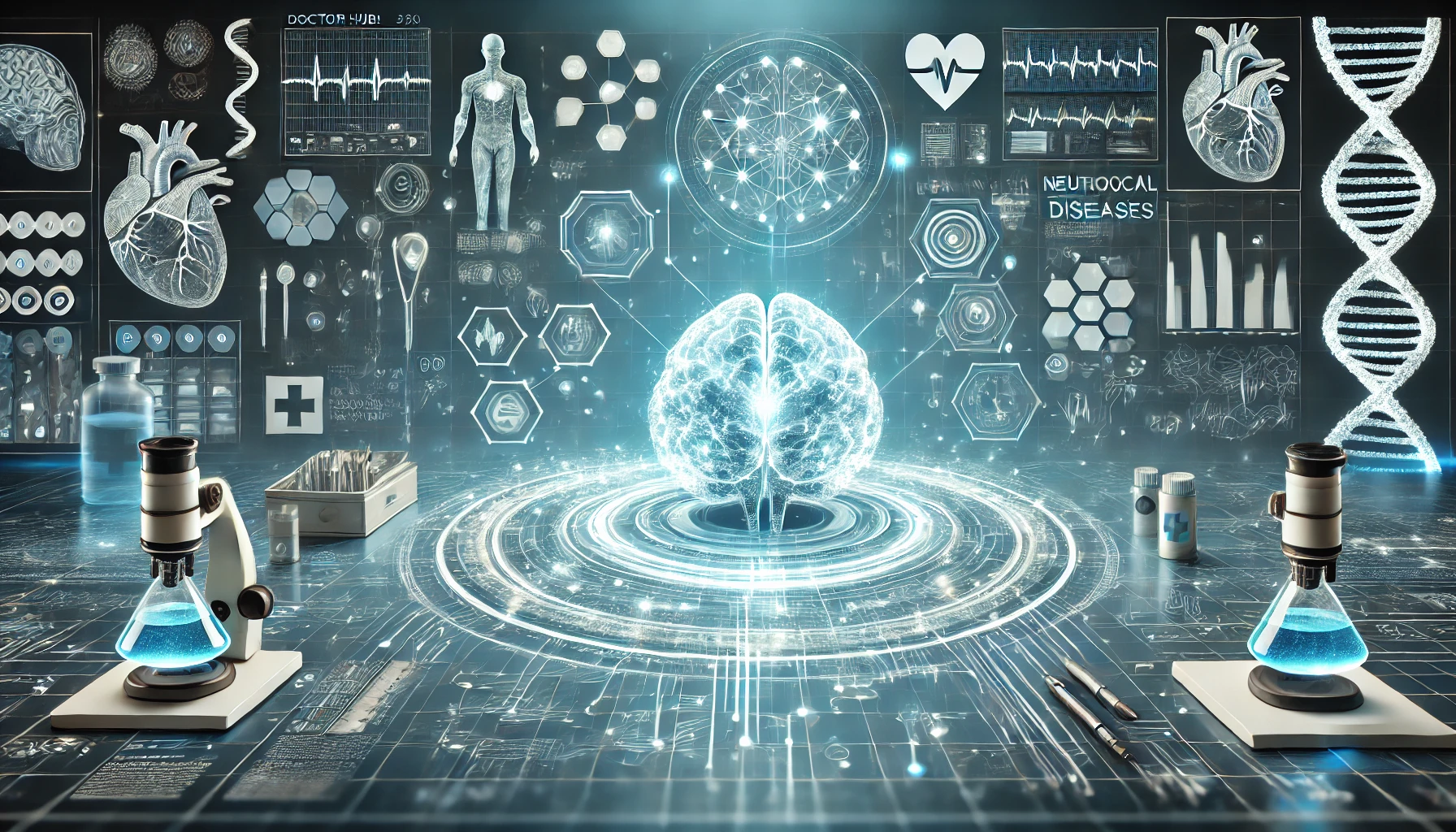
Doctorhub360.com is an advanced healthcare platform designed to support patients suffering from neurological diseases. It uses modern technology to provide medical consultations, symptom tracking, and valuable educational resources. The platform aims to improve patient care and make neurological healthcare more accessible.
Neurological diseases affect the brain, spinal cord, and nerves, leading to severe health complications. Early detection and treatment can significantly improve a patient’s quality of life. Doctorhub360.com neurological diseases platform offers solutions that help in timely diagnosis and management.
With an increasing number of neurological disorders worldwide, there is a growing need for specialized healthcare services. Many patients struggle to access neurologists due to location constraints. Doctorhub360.com helps bridge this gap by providing remote consultations with experts.
The platform is also beneficial for caregivers and family members. It provides guidance on how to care for patients suffering from neurological conditions, ensuring they receive the best possible support.
Understanding Neurological Diseases
Neurological diseases are disorders that impact the brain, nerves, and spinal cord. These conditions can cause symptoms such as muscle weakness, tremors, cognitive decline, and loss of coordination. Some diseases progress slowly, while others cause sudden health crises.
There are several types of neurological disorders, each affecting individuals differently. Some common ones include:
- Alzheimer’s Disease – Causes memory loss and cognitive decline, mostly affecting older adults.
- Parkinson’s Disease – Leads to tremors, muscle stiffness, and slow movements.
- Epilepsy – A condition characterized by frequent seizures due to abnormal brain activity.
- Multiple Sclerosis (MS) – Affects the central nervous system, leading to mobility and vision problems.
- Migraine – A severe headache disorder with nausea and sensitivity to light and sound.
Many neurological conditions have no cure, but treatments can help manage symptoms. Early intervention is essential to slow down disease progression. Doctorhub360.com neurological diseases resources help patients learn about their condition and find appropriate treatments.
Patients need ongoing monitoring and lifestyle adjustments to cope with neurological diseases. Regular consultations with healthcare professionals and tracking symptoms help in better disease management.
How Doctorhub360.com Helps in Neurological Care
Doctorhub360.com provides remote medical consultations for neurological diseases. Patients can connect with neurologists through online appointments, eliminating the need to travel. This feature is especially useful for people living in remote areas.
The platform offers extensive educational resources. Patients and caregivers can access articles, videos, and research-based guides on neurological disorders. These resources help individuals understand symptoms, treatment options, and lifestyle changes.
For effective treatment, tracking symptoms is crucial. Doctorhub360.com offers digital tools that allow patients to log daily experiences. This data helps doctors analyze symptoms over time and adjust treatments accordingly.
Support from others facing similar challenges can be helpful. The platform provides access to community forums where patients and caregivers can share experiences. These support groups offer emotional encouragement and practical advice.
Doctorhub360.com neurological diseases services also incorporate AI-driven recommendations. Artificial intelligence analyzes symptoms and provides personalized health tips, medication reminders, and alerts for potential disease progression.
Benefits of Using Doctorhub360.com
One of the main advantages of Doctorhub360.com is accessibility. Many patients face difficulties visiting hospitals due to mobility issues or long distances. With telemedicine services, they can consult specialists from home.
The platform is cost-effective compared to traditional in-person neurology consultations. It reduces expenses related to hospital visits, travel, and accommodation, making healthcare more affordable.
Doctorhub360.com neurological diseases management tools provide personalized care. AI-driven symptom tracking helps detect changes in a patient’s condition early. This proactive approach improves treatment outcomes.
For caregivers, the platform offers essential guidance on patient care. Neurological disorders often require long-term support, and having the right information helps in managing daily challenges.
Additionally, the platform fosters a supportive community. Patients can join discussion groups, seek advice, and find encouragement from others facing similar conditions. This peer support plays a significant role in improving mental well-being.
Future of Neurological Healthcare with Technology
Technology is transforming the way neurological diseases are diagnosed and treated. AI and machine learning are now being used to predict disease progression and suggest treatment plans. These advancements can help in early detection and better patient management.
Wearable health devices are becoming popular for monitoring brain activity. These devices track neurological signals and detect abnormalities, providing real-time data to doctors. Such innovations make it easier to manage diseases like epilepsy and Parkinson’s.
Doctorhub360.com continues to integrate new technologies into its platform. AI-based recommendations, telehealth solutions, and data-driven healthcare approaches improve neurological care. These advancements ensure that patients receive the best possible treatment.
Hospitals and research institutions are collaborating with online platforms to enhance neurological healthcare. These partnerships allow doctors to share knowledge, access better treatment protocols, and improve patient outcomes.
The future of neurological healthcare is evolving with digital health solutions. Platforms like Doctorhub360.com neurological diseases services are playing a crucial role in making advanced care more accessible to patients worldwide.
Conclusion
Doctorhub360.com provides an innovative approach to managing neurological diseases. By integrating telemedicine, digital health tools, and AI-based solutions, the platform improves access to specialized care.
Understanding neurological diseases is essential for effective management. Patients, caregivers, and healthcare providers can benefit from the educational materials available on Doctorhub360.com. These resources simplify complex medical information, making it easier to understand.
Remote healthcare is becoming more important in modern medicine. Doctorhub360.com neurological diseases services offer convenient, affordable, and personalized solutions for patients struggling with neurological disorders.
As technology continues to advance, online healthcare platforms will play a bigger role in neurological care. Doctorhub360.com remains at the forefront of this transformation, providing reliable and innovative services.
By combining expert medical advice with AI-driven tools, Doctorhub360.com is revolutionizing neurological healthcare. Patients now have better access to information, treatment options, and community support, helping them lead healthier lives.
FAQs
What is Doctorhub360.com, and how does it help with neurological diseases?
Doctorhub360.com is an online healthcare platform that provides telemedicine, symptom tracking, and educational resources for neurological disease management.
Can I consult a neurologist through Doctorhub360.com?
Yes, the platform offers remote consultations with neurology specialists, allowing patients to receive expert advice from the comfort of their homes.
Does Doctorhub360.com provide symptom tracking tools for neurological diseases?
Yes, it includes digital tools for logging symptoms, helping doctors analyze disease progression and adjust treatments accordingly.
Is Doctorhub360.com suitable for caregivers of neurological patients?
Absolutely, it provides caregiving guides, educational resources, and support forums to help caregivers manage patient needs effectively.
How does AI technology improve neurological disease management on Doctorhub360.com?
AI analyzes patient data, offers personalized health recommendations, and alerts users to potential symptom changes for better treatment planning.
HEALTH
Prostavive Colibrim: A Natural Prostate Health Supplement
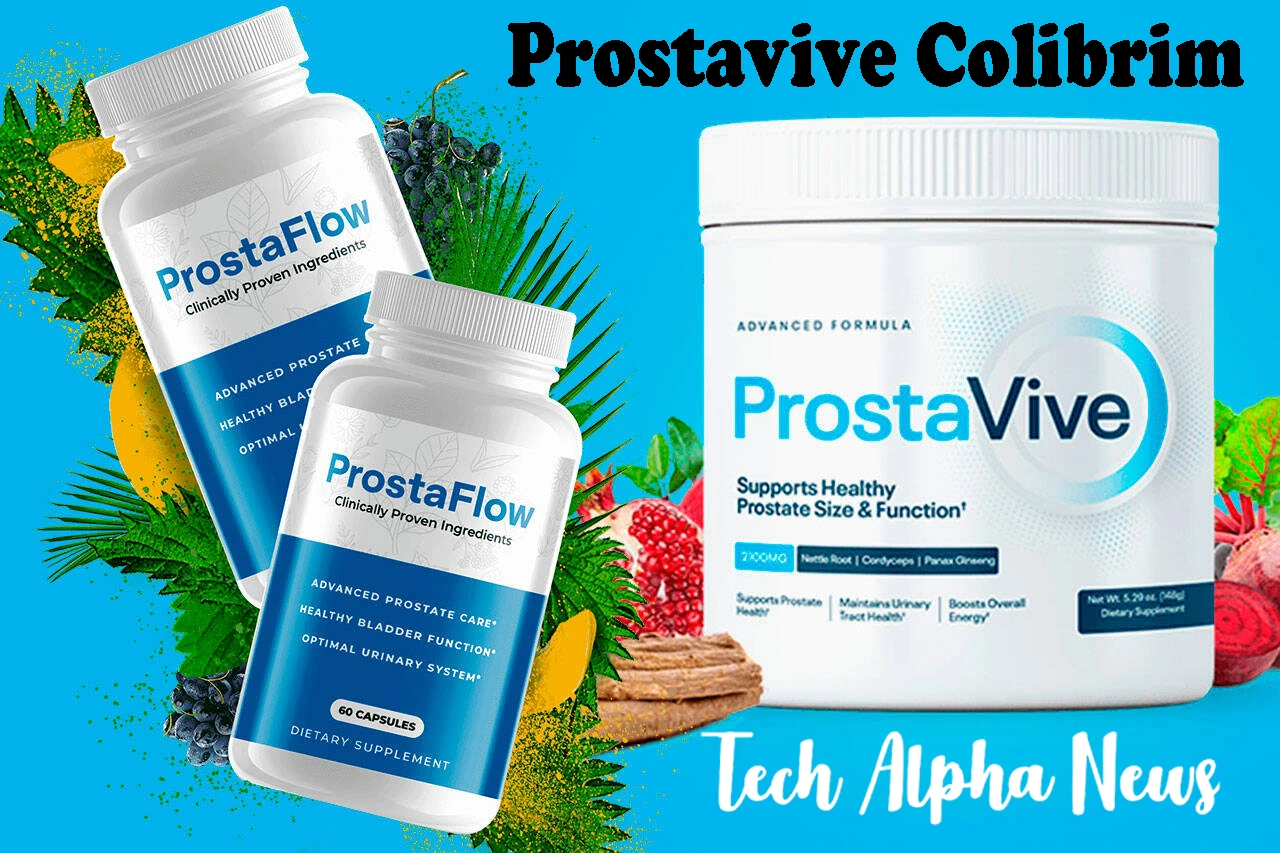
Prostate health is essential for men, especially as they age. Many men experience urinary issues and discomfort due to prostate enlargement. This condition can cause frequent urination, weak urine flow, and other discomforts.
Prostavive Colibrim is a dietary supplement designed to support prostate health naturally. It contains a blend of powerful ingredients that help reduce inflammation and improve urinary function. Many men turn to natural supplements like this to avoid invasive medical procedures.
By maintaining a healthy prostate, men can experience better urinary control and improved quality of life. Prostavive Colibrim aims to provide relief from common prostate-related problems. Regular use may help restore comfort and confidence.
What is Prostavive Colibrim?
Prostavive Colibrim is a supplement made with natural ingredients that support prostate health. It is specifically formulated for men who experience urinary discomfort and prostate enlargement. The supplement works by reducing inflammation and improving urinary flow.
Unlike prescription medications, Prostavive Colibrim is made from herbal extracts, vitamins, and minerals. It is designed to be a safe, non-invasive solution for prostate health. The supplement is available without a prescription, making it easily accessible.
Many users choose Prostavive Colibrim as a natural alternative to traditional treatments. It is commonly used to reduce frequent nighttime urination and other prostate-related symptoms. By using it consistently, men may notice improvements in their overall well-being.
Key Ingredients and Their Benefits
Prostavive Colibrim contains several key ingredients known for their benefits to prostate health. One of the main ingredients is Saw Palmetto Extract, which helps reduce prostate swelling and improves urinary flow. This herb is widely used in prostate supplements.
Another important ingredient is Beta-Sitosterol, a plant-based compound that improves urinary symptoms related to an enlarged prostate. Studies suggest that it helps reduce frequent urination and supports normal urine flow.
Pygeum Africanum is a tree bark extract that has anti-inflammatory properties. It helps improve bladder function and reduces discomfort during urination. Zinc is also included in the formula, as it plays a crucial role in prostate health and immune function.
Pumpkin Seed Extract is another powerful ingredient in Prostavive Colibrim. It is rich in antioxidants and essential nutrients that support prostate wellness. These ingredients work together to promote a healthy prostate and better urinary control.
How Does Prostavive Colibrim Work?
Prostavive Colibrim works by addressing the root causes of prostate discomfort. It helps reduce prostate swelling, which can relieve pressure on the bladder and improve urinary flow. This makes it easier for men to empty their bladder completely.
The supplement also has anti-inflammatory properties that may help reduce discomfort. By lowering inflammation, it can prevent further prostate enlargement and improve overall urinary health. This is particularly important for men experiencing frequent urination at night.
Another way Prostavive Colibrim works is by supporting hormone balance. Some ingredients help regulate DHT levels, a hormone linked to prostate growth. By managing hormone levels, the supplement may prevent further prostate enlargement.
With consistent use, Prostavive Colibrim may lead to better prostate function and fewer urinary problems. It is designed to be a long-term solution for men who want to maintain prostate health naturally.
Benefits of Using Prostavive Colibrim
One of the biggest benefits of Prostavive Colibrim is that it helps reduce frequent nighttime urination. Many men struggle with waking up multiple times at night to urinate, which affects their sleep quality. This supplement may help improve bladder control.
It also supports stronger urine flow, reducing the discomfort of weak or interrupted urination. This can make daily activities more comfortable and less stressful. A stronger urine flow means less straining and a more complete bladder emptying.
Another key benefit is its anti-inflammatory effect, which helps prevent prostate swelling from getting worse. By reducing inflammation, men may experience long-term relief from discomfort and pressure.
Since Prostavive Colibrim contains natural ingredients, it is considered a safe alternative to prescription medications. It provides a non-invasive option for men who want to manage prostate health without medical procedures.
Potential Side Effects and Precautions
Prostavive Colibrim is made from natural ingredients, so it is generally considered safe. Most users do not experience serious side effects when taking the supplement as directed. However, some people may have mild digestive discomfort.
In rare cases, some individuals may have allergic reactions to certain herbal extracts. If a person experiences any unusual symptoms, they should stop using the supplement and consult a doctor. It’s always important to check for possible allergies before trying a new supplement.
Men who are already taking prescription medications for prostate health should speak with a doctor before using Prostavive Colibrim. Some ingredients may interact with other medications. Consulting a healthcare provider ensures safe use.
Although Prostavive Colibrim is designed to be safe, proper dosage and consistency are key. Taking more than the recommended amount will not speed up results and may lead to unwanted side effects.
How to Use Prostavive Colibrim
The recommended dosage for Prostavive Colibrim is usually one or two capsules per day. It should be taken with water, preferably with a meal to enhance absorption. Following the correct dosage ensures the best possible results.
For optimal benefits, users should take the supplement consistently. Natural supplements work gradually, so it may take a few weeks to notice significant improvements. Skipping doses may slow down progress.
To maximize effectiveness, it is helpful to maintain a healthy lifestyle. Eating a balanced diet rich in fruits, vegetables, and lean proteins can support prostate health. Drinking enough water and avoiding excessive caffeine or alcohol may also help.
Regular check-ups with a doctor are still important, even when using natural supplements. A medical professional can monitor progress and provide additional guidance on maintaining prostate health.
Customer Reviews and Effectiveness
Many men who have used Prostavive Colibrim report positive improvements in their urinary health. They often mention fewer nighttime bathroom trips and better urine flow. Some users say they feel more comfortable throughout the day.
However, results can vary from person to person. Some men notice improvements within a few weeks, while others may take longer. The supplement works best when taken regularly and combined with a healthy lifestyle.
There are also some mixed reviews. While many people find relief, others may not experience the same level of benefit. This is common with natural supplements, as individual responses differ.
Overall, Prostavive Colibrim has received mostly positive feedback. Many users appreciate its natural formula and the fact that it does not have harsh side effects.
Where to Buy and Pricing
Prostavive Colibrim is available online through official websites and health supplement stores. Buying from the official website ensures authenticity and product quality. Some retailers may also sell it at discounted prices.
Prices vary depending on the package size. Many sellers offer discounts for bulk purchases, which can help users save money. Some brands also provide a money-back guarantee if users are not satisfied.
When purchasing supplements online, it is important to check for customer reviews and official seller information. This helps avoid counterfeit products that may not be effective.
For the best deals, users can look for special promotions or subscription options. Some websites offer automatic shipments to ensure users never run out of their supply.
HEALTH
Vicki Naas Frederick MD: Advancing Medical Care and Community Development
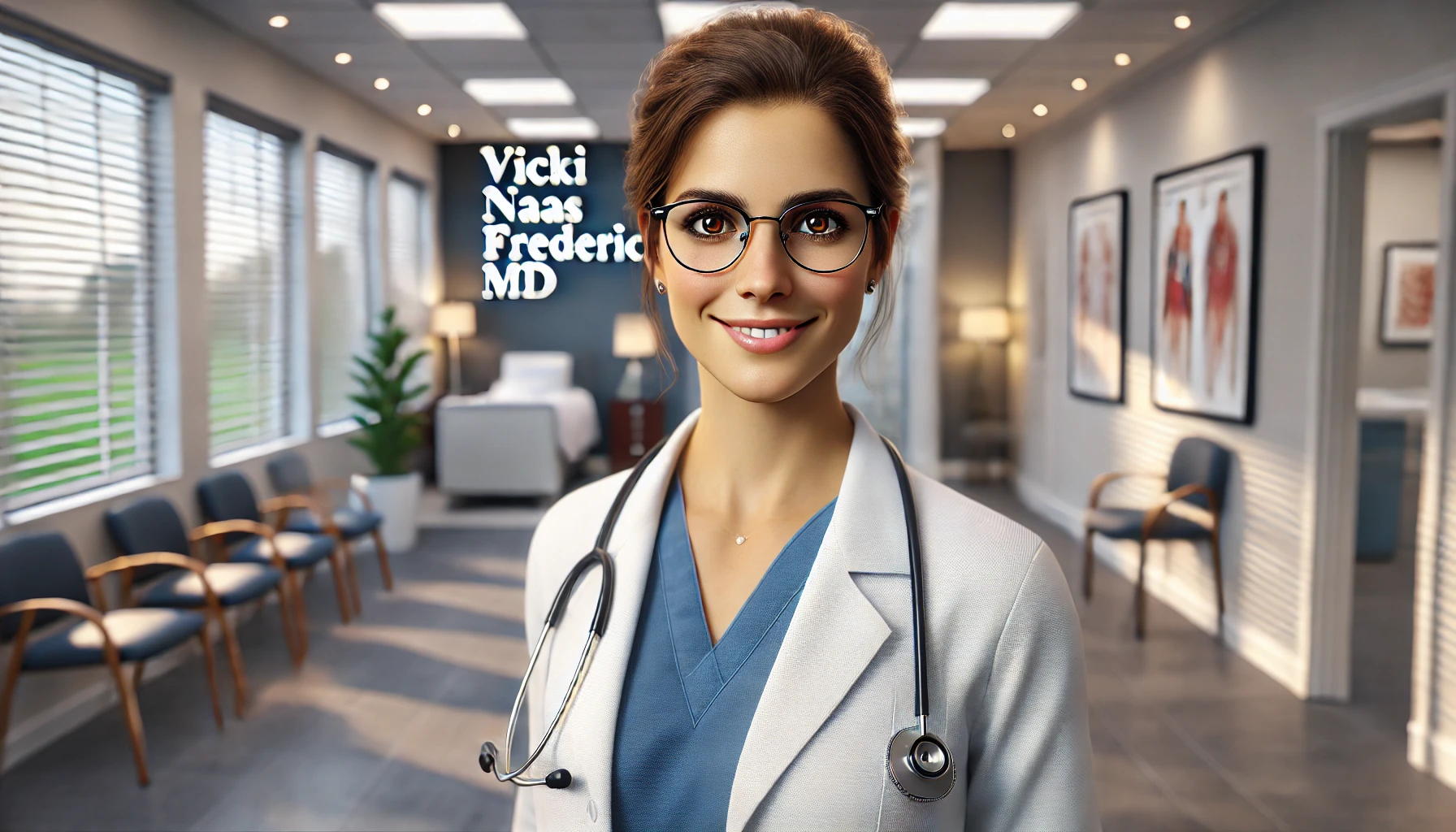
Vicki Naas is a well-known professional and community member in Frederick, Maryland. She has contributed to various fields, including healthcare and business leadership.
Her work focuses on improving healthcare services and economic growth in the region. She has played a significant role in expanding opportunities for residents.
Beyond her professional achievements, she is recognized for her community involvement. She supports nonprofit organizations and works toward educational and social betterment.
Her commitment to innovation and leadership has made a lasting impact on Frederick. The name vicki naas frederick md is associated with progress and dedication in both business and healthcare.
Who is Vicki Naas – Frederick, MD?
Vicki Naas is a well-known professional in Frederick, Maryland, recognized for her contributions to healthcare, business, and community development. She has played a significant role in improving healthcare access, promoting economic growth, and supporting local initiatives that benefit residents.
Her work spans multiple fields, including serving as a family physician, leading a tech startup, and advocating for better healthcare policies. The name vicki naas frederick md is associated with dedication, leadership, and a commitment to making a positive impact in the community.
Background and Personal Life
Vicki Naas has deep roots in Frederick, Maryland. She has lived in the area for many years and is a homeowner on Waterford Drive.
She was born on November 27, 1952, and has Scandinavian heritage. Her background has influenced her strong work ethic and commitment to excellence.
Frederick has been her primary residence, where she has established strong community connections. Her involvement in local events and activities shows her dedication to the town.
Her financial stability reflects her steady career growth. Estimates suggest her net worth is between $50,000 and $99,999, with an annual income of approximately $55,000 to $59,999.
Professional Career
Vicki Naas has made a mark as a family physician in Frederick, MD. She specializes in preventive healthcare and chronic disease management.
Her approach to patient care is focused on compassion and personalized treatment. She believes in building strong relationships with her patients to ensure better health outcomes.
She has been actively involved in promoting telemedicine services. This has improved healthcare accessibility for many individuals in Frederick and beyond.
Her leadership in the medical field has helped shape modern healthcare practices. She advocates for better policies and technological advancements in the industry.
Business and Leadership Roles
In addition to her medical career, Vicki Naas has held leadership roles in business. She served as the CEO of a tech startup, bringing innovative solutions to Frederick’s economy.
Her initiatives have contributed to job creation and local economic development. She has supported businesses in adapting to new market trends and technology.
She focuses on mentoring young professionals and fostering inclusive workplaces. Her leadership style emphasizes collaboration and empowerment.
The growth of businesses under her guidance has strengthened Frederick’s workforce. She continues to support strategic initiatives that boost economic stability.
Table: Economic Contributions of Vicki Naas
Category | Impact |
Job Creation | Expanded employment opportunities |
Business Growth | Helped startups gain stability |
Economic Policies | Advocated for improved strategies |
Workforce Development | Mentored young professionals |
Community Involvement
Vicki Naas is actively involved in various community projects. She supports educational initiatives that provide better learning opportunities for children.
She volunteers with nonprofit organizations aimed at reducing poverty. Her efforts include funding and supporting community-based development programs.
She also advocates for healthcare access for underserved populations. Her work has improved medical services for low-income families in Frederick.
Through partnerships with local businesses, she has helped create pathways for career growth. Her goal is to uplift the community through education and job opportunities.
Financial Overview
Her financial standing reflects her steady career growth and contributions. She has invested in real estate and local businesses in Frederick.
Her estimated annual income is between $55,000 and $59,999. This allows her to maintain a comfortable lifestyle while contributing to the community.
Her net worth ranges between $50,000 and $99,999. This estimate includes property ownership and business investments.
She strategically manages her finances to support long-term projects. Her investments aim to create sustainable economic opportunities in Frederick.
Legacy and Impact
Vicki Naas has built a strong legacy in both the healthcare and business sectors. Her contributions have shaped Frederick’s economic and social landscape.
Her dedication to inclusivity and empowerment has left a lasting impression. She believes in fostering leadership skills among young professionals.
Her name is associated with innovation, healthcare advancements, and economic progress. The impact of vicki naas frederick md continues to inspire many in the community.
Through her work, she has set a foundation for future development. Her commitment ensures that Frederick remains a hub for growth and success.
Conclusion
Vicki Naas has made a lasting impact on Frederick, Maryland, through her dedication to healthcare, business leadership, and community service. Her efforts in patient care, job creation, and economic development have improved the lives of many residents. She continues to advocate for better healthcare access and supports initiatives that promote growth and stability.
The name vicki naas frederick md is recognized for its contributions to progress and innovation. Whether through her medical expertise, business leadership, or philanthropic efforts, she remains a key figure in the community. Her legacy reflects a commitment to positive change, inspiring others to follow in her footsteps.
Her influence extends beyond her professional roles, as she remains actively engaged in making Frederick a better place. Through her ongoing efforts, vicki naas frederick md continues to be a driving force for development and success in the region.
FAQs
Who is Vicki Naas in Frederick, MD?
Vicki Naas is a respected professional known for her work in healthcare, business leadership, and community service in Frederick, Maryland.
What is Vicki Naas’s professional background?
She is a family physician specializing in preventive healthcare and chronic disease management, along with experience in business leadership.
How has Vicki Naas contributed to Frederick’s economy?
She has supported job creation, led a tech startup, and helped businesses grow through innovation and mentorship.
What community initiatives is Vicki Naas involved in?
She volunteers with nonprofits, supports education programs, and advocates for better healthcare access in underserved areas.
Why is Vicki Naas well-known in Frederick, MD?
Her dedication to healthcare, economic development, and community improvement has made her a key figure in Frederick.
-
CRYPTO2 years ago
The Essential Cryptocurrency Laws By State
-
BUSINESS2 years ago
The Evolution of the Patagonia Logo: A Look at the Brand’s Iconic Emblem
-
SPORTS2 years ago
club america vs deportivo toluca f.c. timeline
-
HEALTH1 month ago
Prostavive Colibrim: A Natural Prostate Health Supplement
-
TECH2 years ago
Build Your Email Marketing Contact
-
EDUCATION1 month ago
Ashley Fontera Sterling Middle School: Transforming Education Through Innovation
-
L,IFESTYLE1 month ago
Venus Lusk: The Name That Spans Generations, From Historical Records to Modern Impact
-
CRYPTO2 years ago
Features of Liquidity Providers and Differences Between Them