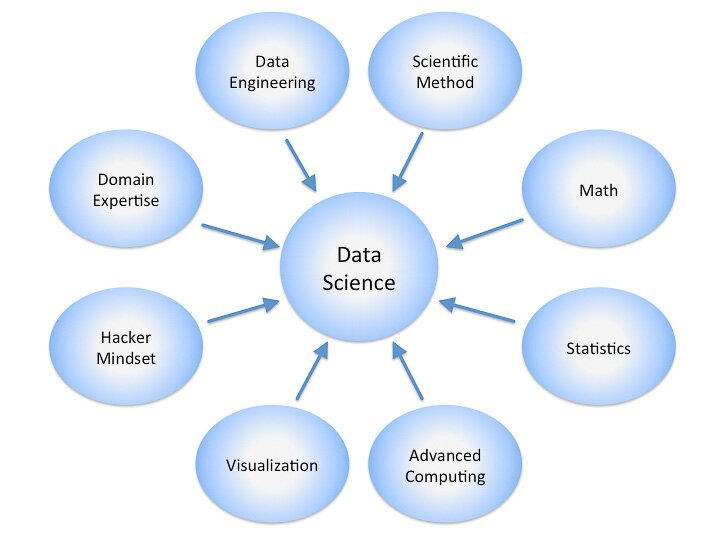
The wide field of records science is concerned with deriving knowledge and insights from organized and unstructured information. It blends domain-specific knowledge with skills from computer science and data to examine, understand, and present complex statistics sets.
Students who enroll in a data science course get an understanding of fundamental concepts like machine learning, programming, and data visualization.
These programs supply students with the necessary equipment to resolve problems, find patterns in big datasets, and make knowledgeable judgments. Anyone interested in a career in this fascinating field should take the Data Science course.
The need for workers proficient in technology is growing as more organizations rely on projects driven by data.
Major Components of Data Science
Among many other academic disciplines, computer programming, statistics, machine learning, and data visualization are all included in the large field of data science. It involves determining the importance of the data.
Every procedure stage is covered, from obtaining data to guarantee accuracy to assessing it to spot patterns and trends. The ultimate goal is to maximize the firm’s reliance on the data.
Enthusiasts can explore the intricacies of this discipline by signing up for a data science course. Let’s look at the key components that comprise the data science framework.
Data Collection and Acquisition
The fundamental component of any Data Science course is data. This first stage is the careful collection of relevant data from various sources. The statistics may also come from semi-structured APIs, unstructured social media posts, or established databases. The capacity to locate, reap, and deal with this information is refined in an intensive path on data science.
Professionals need to understand the nuances of data collection. They learn to recognize biases, evaluate the data’s quality, and select the most effective retrieval methods. The quantity and quality of data are equally important at this stage because the inferences that can be drawn later on are greatly influenced by the data’s integrity.
Data Mining
Data mining is essentially the process of using one or more software tools to evaluate patterns in large volumes of data. It can be applied in many fields, including science and research. Businesses can use data mining to leverage customer information to gain deeper insights, create deeper connections, and optimize resource allocation.
Data Engineering
Data engineering’s primary goal is to create software solutions for data issues requiring the setup of data systems with endpoints and pipelines. Data engineering needs a deep understanding of various data technologies and frameworks and the ability to develop data solutions to support business processes.
Data Visualisation
Effective communication of findings is a crucial competency for a talented data scientist. An essential factor in communicating complex thoughts, understandably is data visualization. Students who enroll in a data science course learn how to use visualization equipment to supply appealing portraits that efficiently convey data-driven narratives.
Choosing the best visualization kind for the statistics and the message you need to explicit is an essential step in generating effective statistics visualization, which goes beyond virtually making visually attractive charts. This capability is important when presenting findings to stakeholders who may not have a technical background.
Big Data Technologies
Data scientists want to be professional at working with big statistics because of the massive variety of information generated these days. Working with frameworks for disbursed computing is a part of this.
Data Science course modules frequently blanket big information technologies, which help students scale their research to manipulate massive datasets successfully.
To completely utilize the available records, one must have a solid knowledge of huge information technology. It opens the door to extra in-depth investigations by enabling data scientists to work with datasets that are more extensive than those supported through conventional computer platforms.
Machine learning
Machine Learning is the data science department that uses distinct algorithms to perform on big volumes of data accrued and extracted from numerous resources, permitting the gadget to deal with datasets autonomously without human intervention.
It forecasts, analyses patterns, and provides advice. Fraud detection and consumer retention are two common applications of machine learning.
A social media platform is a fantastic place to see machine learning in action, as users’ behaviour is gathered using rapid and effective algorithms to offer multimedia files, articles, and much more, depending on their interests.
Machine learning is another branch of artificial intelligence that uses a range of techniques and algorithms to acquire the required knowledge.
Exploratory Data Analysis
After cleaning and preparing the statistics, exploratory data analysis is completed. Finding and understanding the correlations, styles, and traits in the data is the primary goal in this situation.
In a Data Science course, college students research statistical techniques and visualization equipment that are valuable resources in pattern recognition. Analyzing exploratory records is essential as it establishes the foundation for formulating theories and identifying traits that can be huge for modelling.
This is the section where data scientists study the tale the data tells and provide essential context for additional studies.
Artificial Intelligence
The purpose of Artificial intelligence is to allow software programs to observe and examine their environment via machine learning models that perceive patterns or predefined guidelines and search engines, after which they make choices primarily based on those tests.
Artificial intelligence tries to mimic biological intelligence to decrease manual human intervention for several duties. As a result, the system or software can perform with special degrees of autonomy.
Career Opportunities of Data Science Course
Within the ever-evolving field of data science, numerous key positions have developed, all of which can be critical to using data. Leading the charge in extracting actionable insights from complex datasets is the field of data science.
The production of algorithms through Machine learning engineers improves automation and prediction models. The infrastructure required for generation era and storage is built via data engineers. Data is transformed into strategic enterprise selections by business intelligence analysts.
For these roles, a competitive coding course is required. For jobs like statistics scientists and machine learning engineers, one must be talented in algorithms and coding. Professionals may be a part of an aggressive coding school to gain the problem-solving abilities required in a competitive work marketplace.
Enhancing algorithmic reasoning increases performance and productivity in data-driven jobs. Competent coding abilities combined with unique work ensure that human beings remain adaptable and capable in this fast-paced place, especially because the need for data science knowledge increases.
Conclusion
In our data-driven world, data science is the revolutionary force driving insights, innovation, and well-informed decision-making.
A thorough examination of the fundamental ideas, tools and methods needed to successfully navigate the ever-evolving field of data analysis was given in the Data Science course.
This course equips students to succeed in the ever-evolving field of data science and acts as a catalyst for a future powered by data.